Loop the values of a correlated variable
A ForEach controller loops through the values of a set of related variables. When you add samplers (or controllers) to a ForEach controller, every sample (or controller) is executed one or more times, where during every loop the variable has a new value. The input should consist of several variables, each extended with an underscore and a number. Each such variable must have a value. So for example when the input variable has the name inputVar, the following variables should have been defined:
- inputVar_1 = wendy
- inputVar_2 = charles
- inputVar_3 = peter
- inputVar_4 = john
Note: the "_" separator is now optional.
When the return variable is given as "returnVar", the collection of samplers and controllers under the ForEach controller will be executed 4 consecutive times, with the return variable having the respective above values, which can then be used in the samplers.
In this example, we created a Test Plan that sends a particular HTTP Request only once and sends another HTTP Request to every link that can be found on the page.
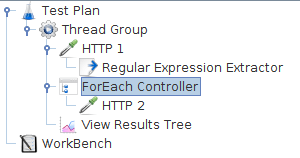
We configured the Thread Group for a single thread and a loop count value of one. You can see that we added one HTTP Request to the Thread Group and another HTTP Request to the ForEach Controller.
After the first HTTP request, a regular expression extractor is added, which extracts all the html links out of the return page and puts them in the inputVar variable
In the ForEach loop, a HTTP sampler is added which requests all the links that were extracted from the first returned HTML page.